
Risk Assessment and Asset Allocation with
Gross Exposure Constraints for Vast Portfolios
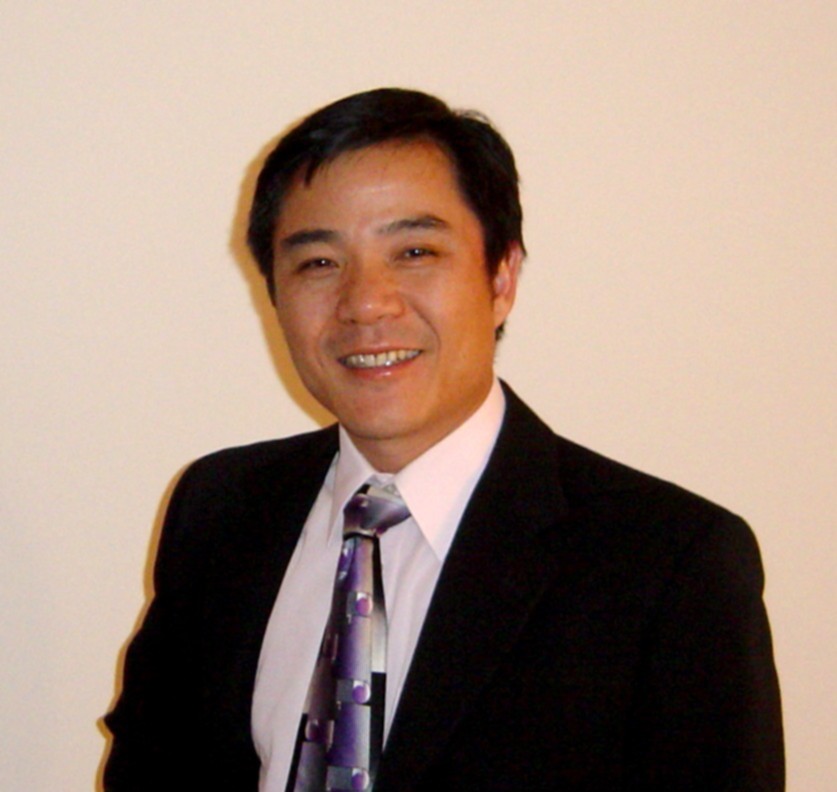
|
Professor
Jianqing Fan
Frederick L. Moore Professor of Finance
Morningside Gold Medal for Applied Mathematics
Humboldt Research Awardee
COPSS Awardee
President of Institute of Mathematical Statistics
Fellow, American Association for Advancement of Science
Fellow, Institute of Mathematical Statistics
Fellow, American Statistical Association
Elected Member, International Statistical Institute
Photo
|
Date: |
18 December 2008 (Thursday) |
Time: |
11:00am
- 12:30 pm (Preceded by Reception at 10:30 am) |
Venue: |
LT2, C. S. Tse Lecture Theatre 2,
Ho Sin Hang Campus,
Hong Kong Baptist University
|
|
|
|
Abstract
Markowitz (1952, 1959) laid down the ground-breaking work on the mean-variance analysis. Under his framework, the theoretical optimal allocation vector can be very different from the estimated one for large portfolios due to the intrinsic difficulty of estimating a vast covariance matrix and return vector. This can result in adverse performance in portfolio selected based on empirical data due to the accumulation of estimation errors. We address this problem by introducing the gross-exposure constrained mean-variance portfolio selection. We show that with gross-exposure constraint the theoretical optimal portfolios have similar performance to the empirically selected ones based on estimated covariance matrices and there is no error accumulation effect from estimation of vast covariance matrices. This gives theoretical justification to the empirical results in Jagannathan and Ma (2003). We also show that the no-short-sale portfolio is not diversified enough and can be improved by allowing some short positions. As the constraint on short sales relaxes, the number of selected assets varies from a small number to the total number of stocks, when tracking portfolios or selecting assets. This achieves the optimal sparse portfolio selection, which has close performance to the theoretical optimal one. Among 1000 stocks, for example, we are able to identify all optimal subsets of portfolios of different sizes, their associated allocation vectors, and their estimated risks. The utility of our new approach is illustrated by simulation and empirical studies on the 100 Fama-French industrial portfolios and the 400 stocks randomly selected from Russell 3000.
|
|
The Medium of Instruction:
English |
All
are welcome |
|
|